One of my predictions for 2024 in the AI world was the creation of more powerful text-to-video models. It’s only the second month of the new year, and OpenAI has announced a new neural network, Sora, which demonstrates incredible results in generating video from text.
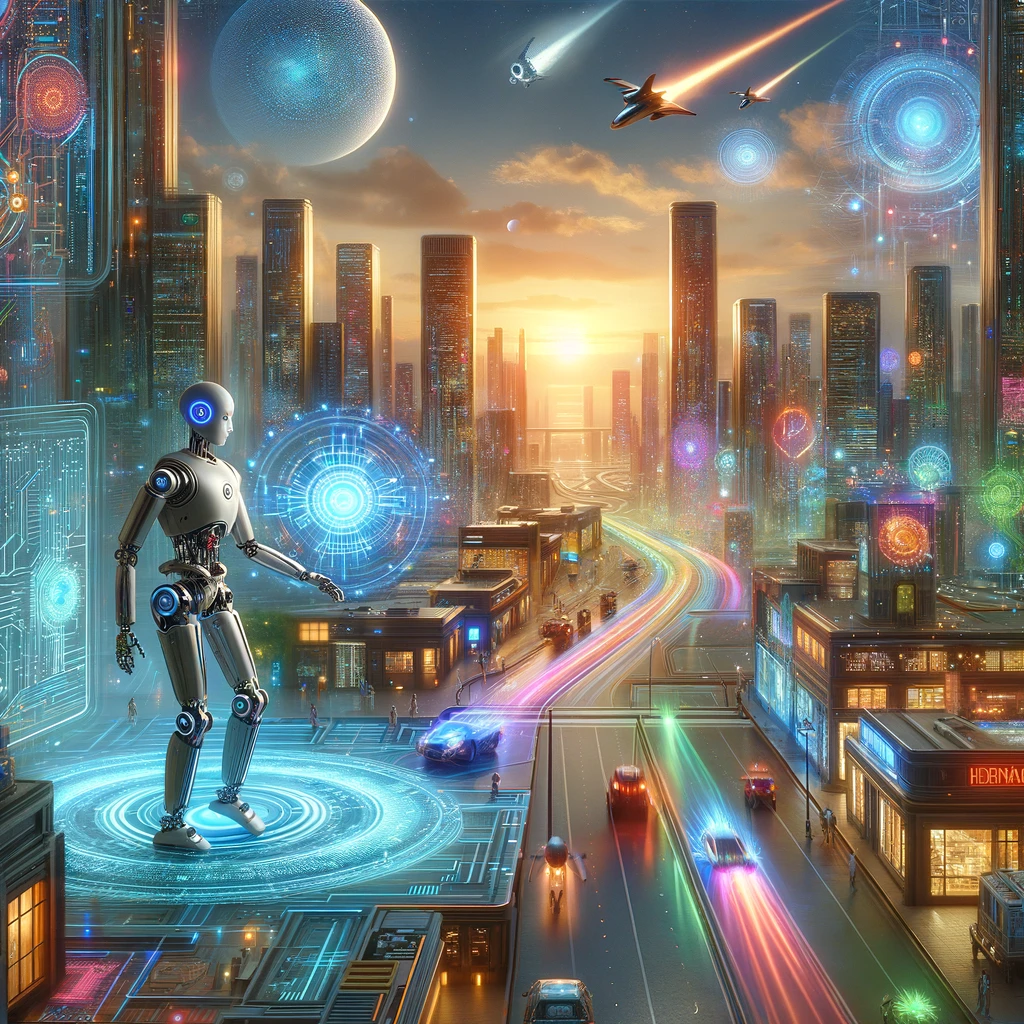
At the moment, Sora is available to a limited number of people, and technical details are not particularly disclosed. However, OpenAI released an article "Video generation models as world simulators", in which they lifted the veil of secrecy. Despite the fact that it does not shine with details, very interesting ideas and thoughts pop up in it. In this article, I will briefly analyze the most interesting points from the work of OpenAI.
Transformer is all you need
To train Sora, all input videos are compressed using a specially trained neural network. Video compression occurs both spatially and temporally. In other words, all videos are converted into a special lower-dimensional latent space. Ultimately, Sora's job is to generate videos in a given space.
After generating a video in a given latent space, it needs to be converted into a "regular video space" so that we can watch it. This happens due to another neural network – the decoder. That is, we have a U-net-like architecture.

Inspired by modern LLM and Vision Transformer, Sora uses Transformer architecture as the basis. After the video has been compressed into a special latent space, it is divided into spacetime patches. This allows for the generation of videos of almost any length and with different resolutions and aspect ratios.
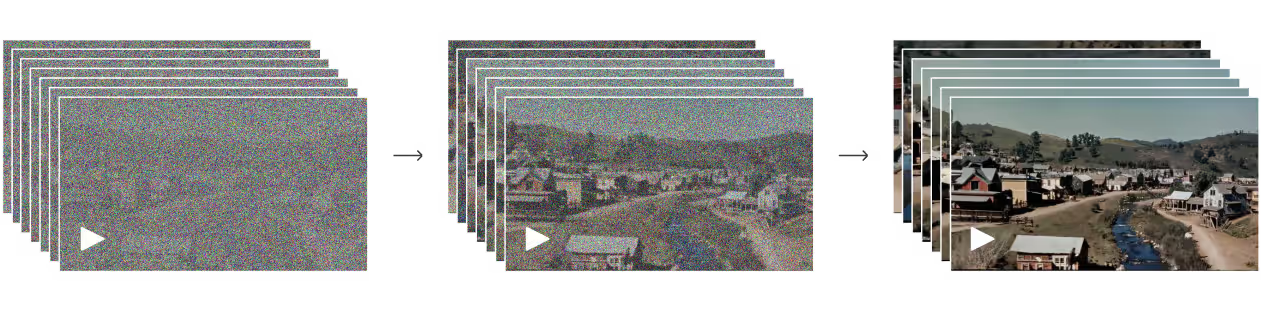
And as you might have guessed, Sora is a diffusion transformer that tries to generate "clean" patches from noisy patches.
Sizes matter
As we know, Transformers scale well. What does this mean in this context? In simple words, the larger the model and the more computing power, the better the result. The authors of Sora showed that their diffusion transformer satisfies this rule when generating video.
Base compute
4x compute
32x compute
Prompts improvements using GPT
To train a text-to-video model, we need a huge amount of videos with well-written text descriptions. There are very few such datasets, so researchers from OpenAI used the same trick that was used in DALL·E 3.
The trick is to train a separate model to write a high-quality but short description, and then use GPT-4 to improve and expand that description. Learning from synthetic descriptions significantly increases the quality of generation, the researchers note.
Sora can do everything (almost)
As mentioned earlier, Sora is based on a transformer that receives vectors and outputs vectors. Therefore, we can do almost everything with the output vectors. For example, we can generate an image, animate an existing image, regenerate or modify a video, or smoothly merge two videos. All this is already working quite well.
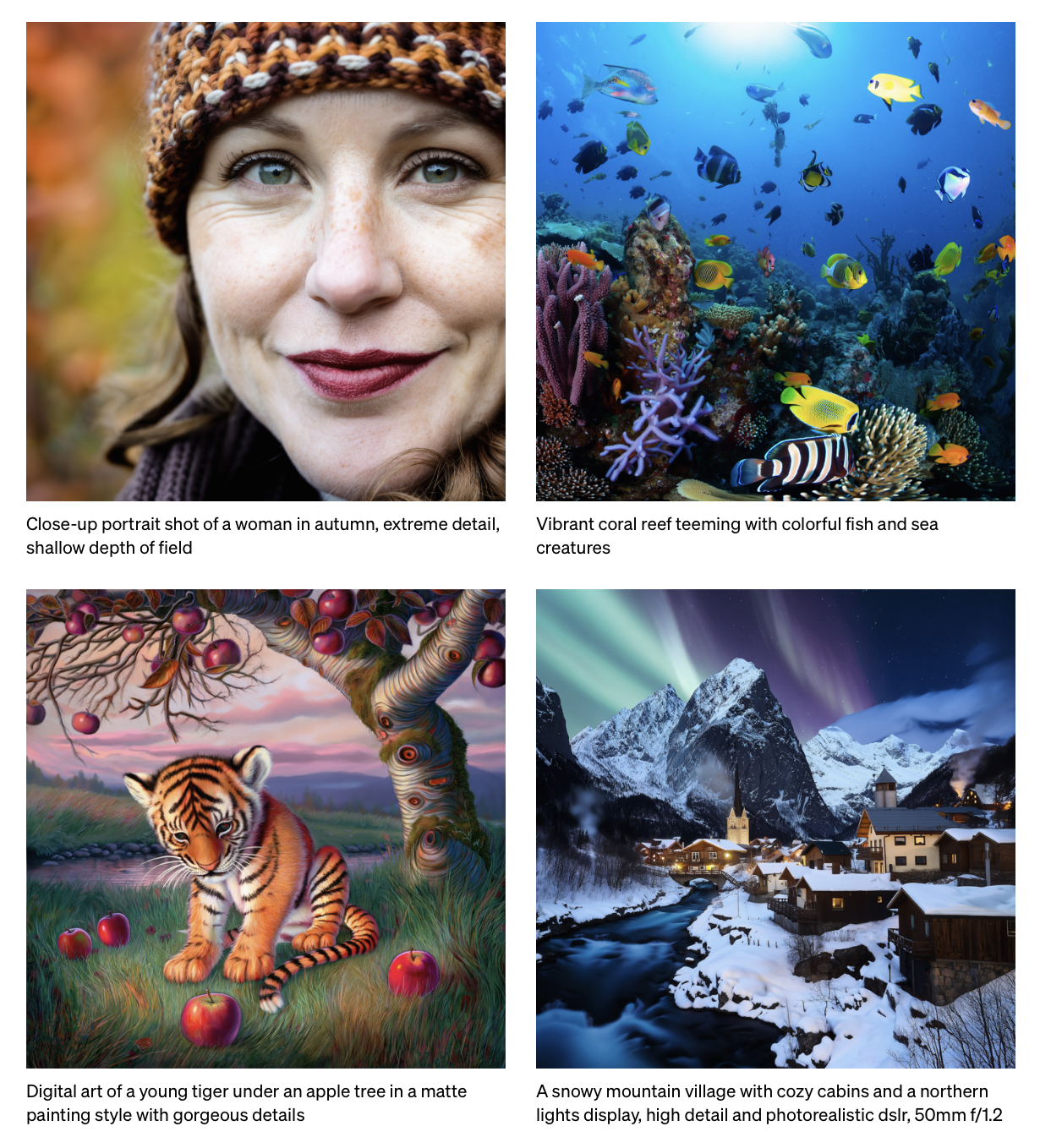
Why is Sora a world simulator?
The OpenAI article has a great title. Let me remind you: "Video generation models as world simulators". Why is Sora a world simulator? Researchers indirectly confirm this very loud name with the help of the following observations.
3D consistency
The camera can move and rotate freely in the "virtual world".
Long-range coherence and object permanence
Throughout the entire video, objects will not change either shape or texture. If some objects disappear from the frame, they often appear the same as they were before the disappearance, and in the correct place.
Interacting with the world
Objects can interact with the world and change realistically.
Simulating digital worlds
Sora can recreate the world of the Minecraft game and simultaneously simulate player behavior, as well as display the world and its changes with high accuracy. So, for example, a player can move around the map, his inventory is consistently displayed below, and the surrounding environment does not change when he changes the direction of view.
Thus, Sora, to some extent, learned to perceive the world almost like a person. Many people have already started talking about AGI. But, in my humble opinion, AGI is still a long way off.
Conclusion
The Sora's demos are very impressive. This is a completely new level in text-to-video generation. On the one hand, such results are very inspiring and give strong hope that this year we will see a lot of powerful work devoted to video generation. On the other hand, the quality of the work of generative neural networks is frightening. Video deepfakes are much more dangerous than image deepfakes. Researchers now need to come up with methods to combat them. The usual watermark at the bottom of the video is clearly not enough...